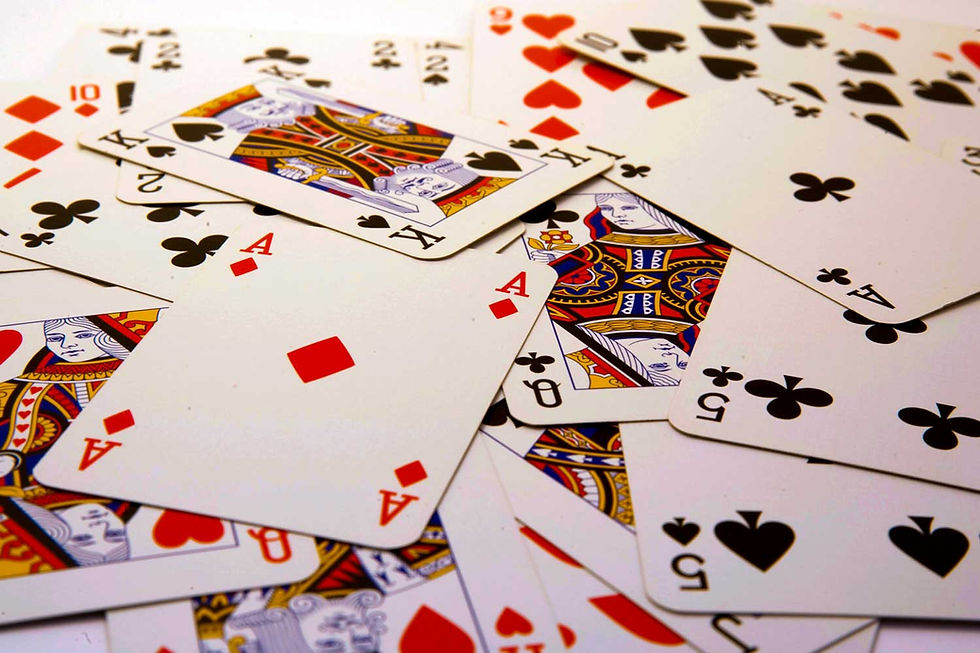
"Most often when teams are being formed in organizations, time availability and resumes/CVs are used as the factors for selection. Those two factors are how teams have been formed for over a century.
An additional method or data point for forming teams is now coming to the forefront, which is including the human dynamics/personality of the different team members.
If you don’t take that into account, it’s like having cards face down in the deck. If you do take take that into account, the cards are face up, and you can orchestrate your team(s) in a more effective way. So you can tailor personalities to goals and desired outcomes."
– Gary Wilhelmi, Idea Connection Systems
Imagine a deck of cards where each card represents an employee. They’re all face down. All you know is you have a deck of playing cards because you see the decorative brand graphics. Everything else is hidden and unknown.
Now imagine the cards are your employees and you’re forming multiple teams by dealing the cards into multiple hands. Being totally random, you’re likely to get a couple of very good teams and whole bunch of not-so-effective teams.
Try again, only this time with the cards all face up and deal out a new array of teams specifically choosing winning hands. Obviously, you’re now going to deal very intentional hands, from straights to royal flushes.
That’s what the Innovation Strengths Preference Indicator® (ISPI) does. It lets you deal with the cards face up. Because you know from each person’s data precisely how they approach problem solving. You can carefully and deliberately group cards for similarity or diversity, whichever is needed.
It’s not only accurate, but also fast enough to allow you to organize different teams on the fly to get the particular results you need.
This is exactly what we demonstrated in our work with a major military university.
Organizing teams for optimum innovation
We were asked to support a “Shark Tank” like project in an elective class by organizing about 70 students into six teams. For this exercise, we put together like minded teams - people who approached problems with the same motivations and mindsets.
We grouped wildly creative “pioneers” in a team and more button-down, analytic “builders” in another group. Also importantly, there were teams that were somewhere in the middle of the scale between builders and pioneers. The teams were similar in their mindset and approach to the assignment.
You can’t build teams like that with only CVs and interviews. That would be working with the “card deck” face down. But with the ISPI™ assessment, the cards are all turned over for easy and fast selection.
We went into this job allowing three days to assign teams. But armed with the data we knew we could trust, we quickly realized assembling a few teams of “high pioneers” or mid-level builders was as easy as picking out cards laying face up on the table.
When we got to the second cohort at the university, we were forming teams in a couple of hours. And by the third cohort, we were in sync with the customer needs and were able to turn it around very quickly.
Using the ISPI we can quickly spot each person’s work style and point of view, to create either highly similar-minded teams or the perfect blend of diversely thinking teams.
The exercise was a success for the university. The class got a hands-on demonstration on how innovation can happen with teams selected according to innovation preferences.
How well did we predict the performance?
Our job was to form the teams for the assignment, according to innovation preferences of the individuals. But there was a second meta component of the project with the university: demonstrate performance predictability.
Faculty wanted to know how accurately we could form teams and how we could forecast how the teams would behave based on how the individuals would work together to meet the challenge.
We knew the ISPI™ would give us this predictability. We’ve been doing this for 35 years. For this university client, we did a post-assignment analysis with the client and agreed that our forecast of how all six teams would perform was 83 percent accurate.
It’s highly unlikely you’d get anywhere close to that if you only used resumes and interviews to form teams. You might as well use a random selection method. If you want the most predictable innovation teams, turn “the cards” face up and choose using the ISPI™ data. For more information on this case study and how it might work for your organization, contact Andrew Harrison.
Comments